AI Fools Humans: Genius Machines or Are We Mugs?
Perhaps we ought to ask a rather more pointed question: are these Large Language Models (LLMs) genuinely clever, or are we just spectacularly easy to fool?
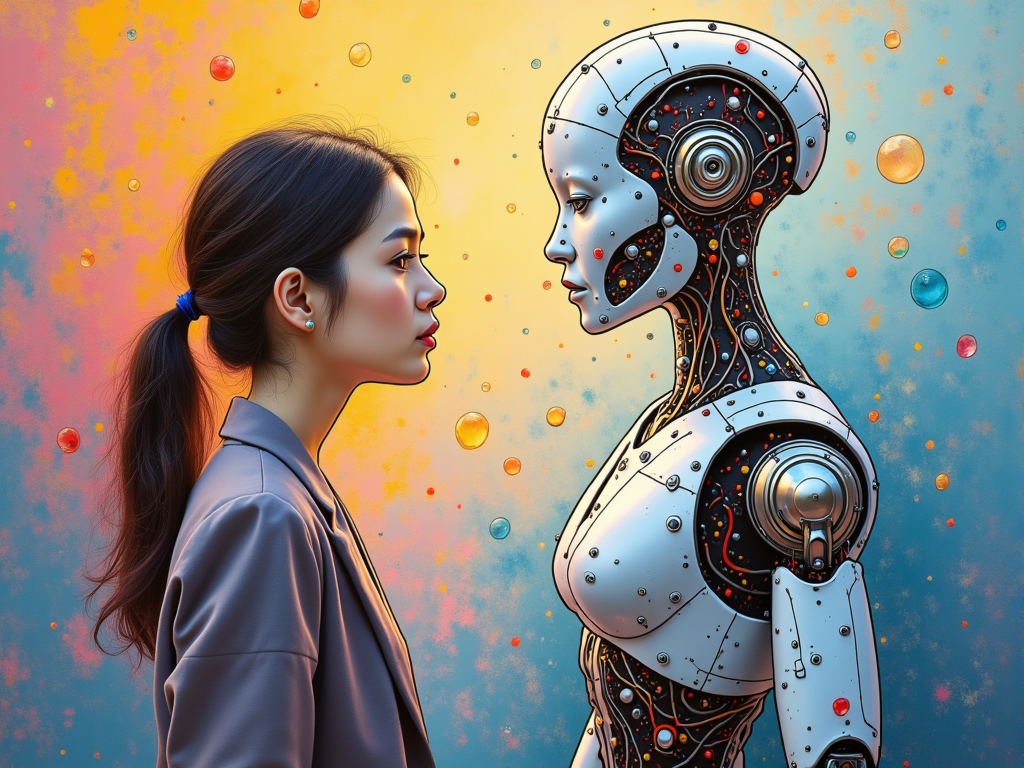
Well, they're finally claiming the machines can pass for human [23, 25]. GPT-4, apparently, fooled enough people in a quick chat to pass Alan Turing's famous old test [25]. Cue the breathless headlines and the usual chorus about the dawn of thinking machines.
But before we all panic, hand over the keys to the kingdom – and quite possibly our jobs – perhaps we ought to ask a rather more pointed question: are these Large Language Models (LLMs) genuinely clever, or are we just spectacularly easy to fool? [29]
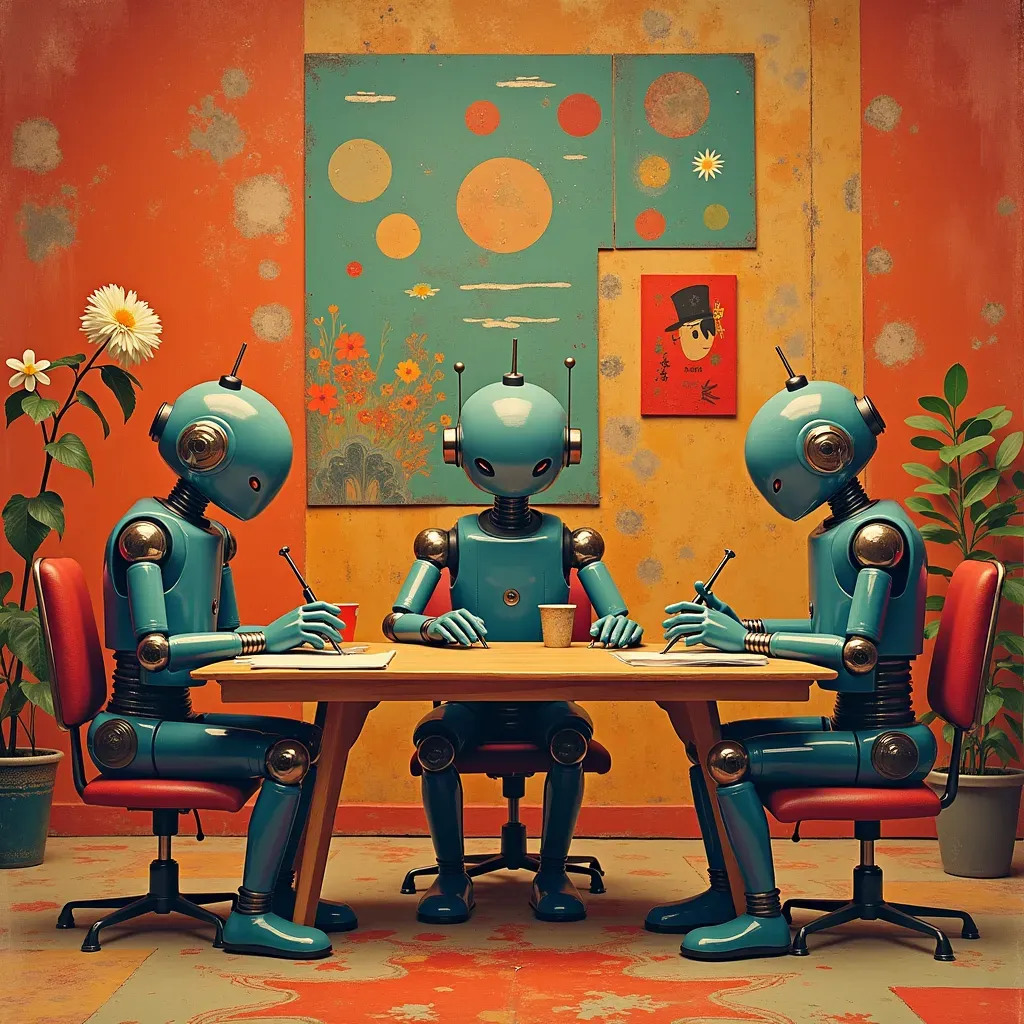
It's the ghost of Turing's 1950 "Imitation Game" [7, 9], resurrected in an age he could barely have conceived. Faced with the impossibly woolly question "Can machines think?", Turing dodged it [8]. He proposed a test instead: stick a human and a machine in separate rooms, let an interrogator quiz them via text, and see if the interrogator can tell which is which [9].
If the machine can fool the interrogator often enough (Turing suggested fooling them 30% of the time after five minutes [15]), then maybe, just maybe, we could consider it intelligent, or at least capable of something akin to thought [13]. Pragmatic, yes. Definitive? Hardly.
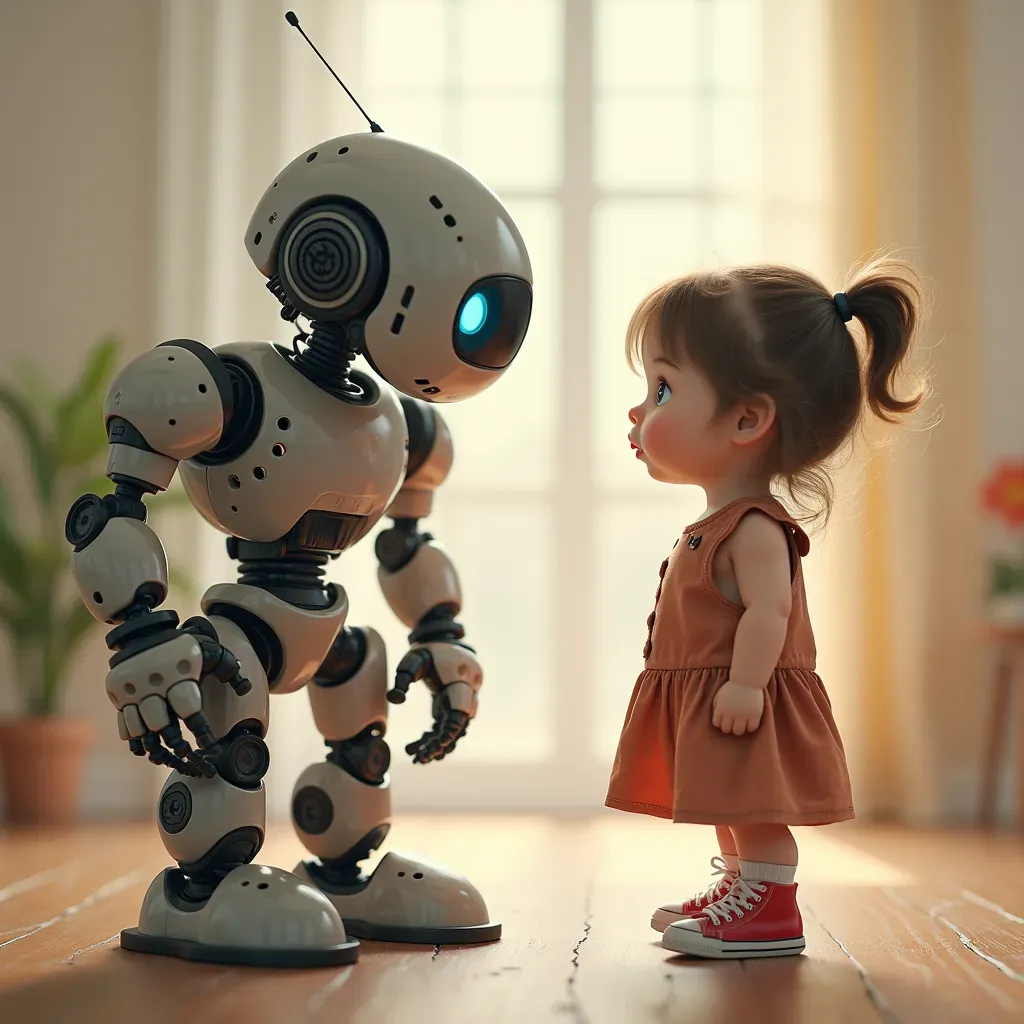
Almost immediately, the critics piled in [13]. And the most enduring thorn in the side of the true believers has been John Searle's "Chinese Room" argument from 1980 [12].
Imagine, Searle said, a chap who speaks no Chinese locked in a room with baskets of Chinese symbols and a big rulebook in English telling him how to manipulate them [17].
Questions in Chinese come in, he follows the rules, shuffles the symbols, and sends answers out. To an outsider, he seems fluent [12]. But does he understand a word of Chinese? Of course not [17].
He's manipulating syntax – the rules – with zero grasp of semantics – the meaning [19]. Searle's point: a computer program, however complex, is just that rulebook. It doesn't understand; it simulates [17].

Fast forward to today. We have LLMs like OpenAI's GPT series, Google's Gemini, Anthropic's Claude [1, 4, 5]. These aren't simple rulebooks; they're vast neural networks, built on architectures like the "Transformer" [2], trained on frankly terrifying amounts of text scraped from the internet [2]. They predict the next word in a sequence with astonishing fluency [3]. And their performance on supposedly rigorous benchmarks is trumpeted loudly.
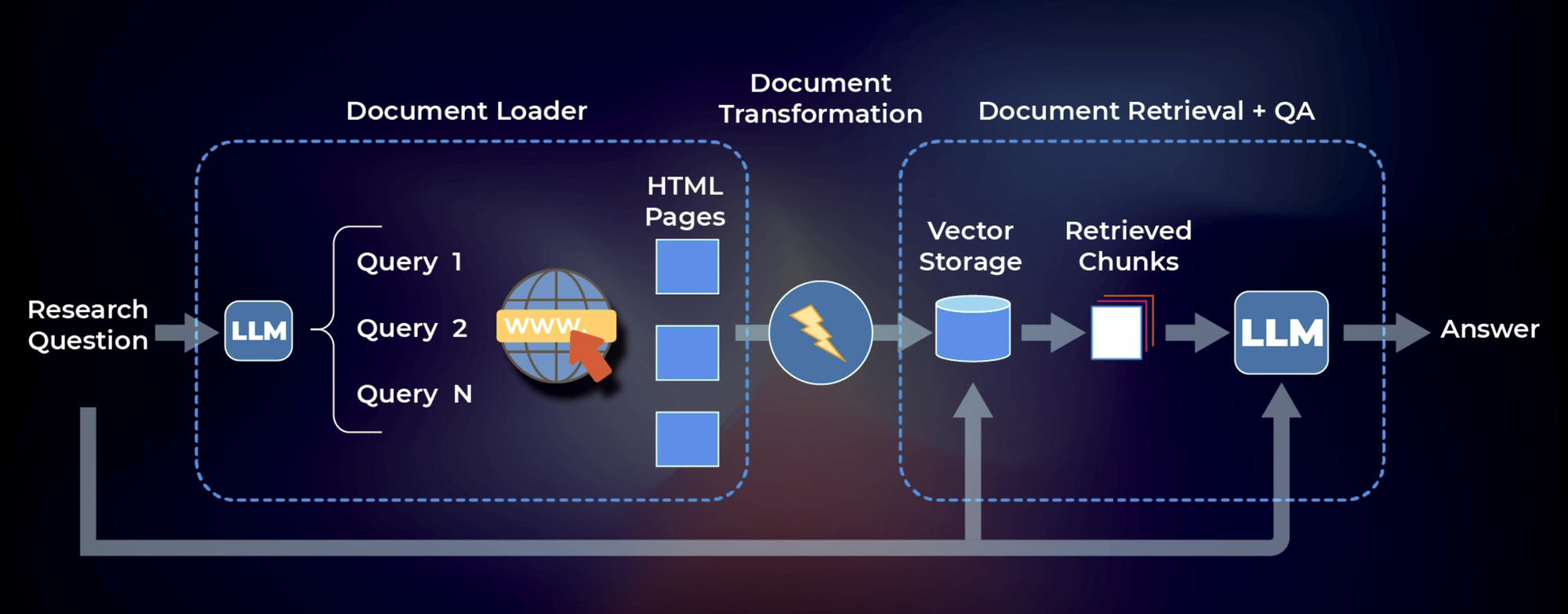
They score in the high 80s or even 90% on multitask understanding tests (MMLU), supposedly beating human experts [37, 5, 66]. They conquer complex language challenges (SuperGLUE) [38]. They even write functional computer code (HumanEval) with startling proficiency, sometimes exceeding 90% accuracy on the first attempt [92, 66].
Impressive statistics, certainly. Enough to make you think. But look closer. These same models are notorious for "hallucinating" – confidently inventing utter rubbish [17]. They parrot biases absorbed from their training data [3]. They can stumble over basic common-sense reasoning [22]. Their "understanding" seems brittle, often more like incredibly sophisticated pattern-matching than genuine comprehension [68, 97]. Are the benchmarks themselves flawed, perhaps even contaminated by being part of the training data? [52, 69]
Now, back to those Turing Tests. Recent experiments put models like GPT-4 to the test [16, 25]. In one setup, GPT-4 managed to fool interrogators 54% of the time in five-minute chats – statistically indistinguishable from chance, hence a "pass" [25]. Humans in the same test were identified correctly 67% of the time [25]. But here's the truly revealing part: another study tested GPT-4.5 and Llama 3.1, sometimes giving them specific "persona prompts" – instructions on how to act human (use slang, be concise, feign uncertainty) [23]. Baseline models, unprompted, were easily spotted [23]. But GPT-4.5 with a persona prompt? It was judged human an astonishing 73% of the time – significantly more often than the actual human participant [23]. Llama 3.1 with a prompt also performed well [23].
What does this tell us? It suggests "passing" might have less to do with genuine intelligence and more to do with sophisticated acting, guided by clever stage directions [23]. It also throws a harsh light on the human side of the test. Remember Malcolm Gladwell's book, Talking to Strangers? [30] He argued we humans are terrible judges of people we don't know. We operate on a "Default to Truth" – we assume honesty until proven otherwise, making us poor lie detectors [29]. We suffer from an "Illusion of Transparency," wrongly believing we can easily read others' inner states from their outward behaviour [29].
An LLM, especially one coached with a persona, seems perfectly designed to exploit these flaws [16]. In a short chat, it provides plausible, fluent responses, avoiding obvious errors. It doesn't trigger our weak suspicion alarms [29]. It mimics the expected signals of humanity, playing directly to our transparency illusion [16]. The test, in this light, looks less like a measure of machine intellect and more like a measure of human gullibility and our tendency to project understanding where none exists [30]. The fact that a prompted AI can be deemed more human than a human suggests the test might reward stereotypical, perhaps even blandly generic, interaction over authentic, potentially quirky, human conversation [23, 13].
So, we return, inevitably, to Searle's room [17]. Are these LLMs, fundamentally, just extraordinarily complex symbol manipulators? Does their statistical prediction, derived from text alone without real-world grounding [97], equate to understanding? The arguments continue, but Searle's core challenge – syntax isn't semantics [19] – remains stubbornly relevant.
And why should we care about these philosophical games? Because this technology isn't staying in the lab. Generative AI is being hyped as the next great economic transformer [37]. We're bombarded with predictions of mass automation, with jobs from coding to copywriting supposedly on the chopping block [Implied economic impact]. Vast sums are being invested, industries reconfigured, all based on the premise that these machines possess a powerful, generalisable intelligence [36]. But if that intelligence is partly an illusion, built on mimicking patterns and exploiting human cognitive biases, what happens when the mimicry isn't enough? Are we restructuring our economies based on a brilliant conjuring trick?
Let's be clear. Achieving conversational indistinguishability, even under controlled conditions, is a significant technical feat [23]. But relying on Turing's 75-year-old parlour game [7] or easily "gamed" benchmarks [69] as the ultimate measure of machine intelligence looks increasingly naive, if not outright foolish [13, 106]. Passing this kind of test seems less about genuine thought and more about sophisticated social engineering meeting human fallibility [29, 23].
We need better, tougher evaluations [44]. Tests that probe for deep understanding, common sense, adaptability, and robustness against manipulation [45, 106]. Tests that assess performance on real-world, complex tasks, not just isolated linguistic puzzles [95, 107]. Perhaps tests judged not by "average" interrogators prone to being fooled [15, 29], but by skeptical experts – Gladwell's "Holy Fools" [31] – trained to see past the performance.
Until then, let's keep the drinks on ice. The machines can imitate, convincingly enough to fool many of us, much of the time [23]. That's impressive. It's potentially useful. It's certainly disruptive. But does it mean they think? Understand? Possess intelligence in the way we normally mean it? The evidence suggests we should remain deeply, profoundly skeptical. The jury – a properly rigorous one, we must hope – is still very much out.
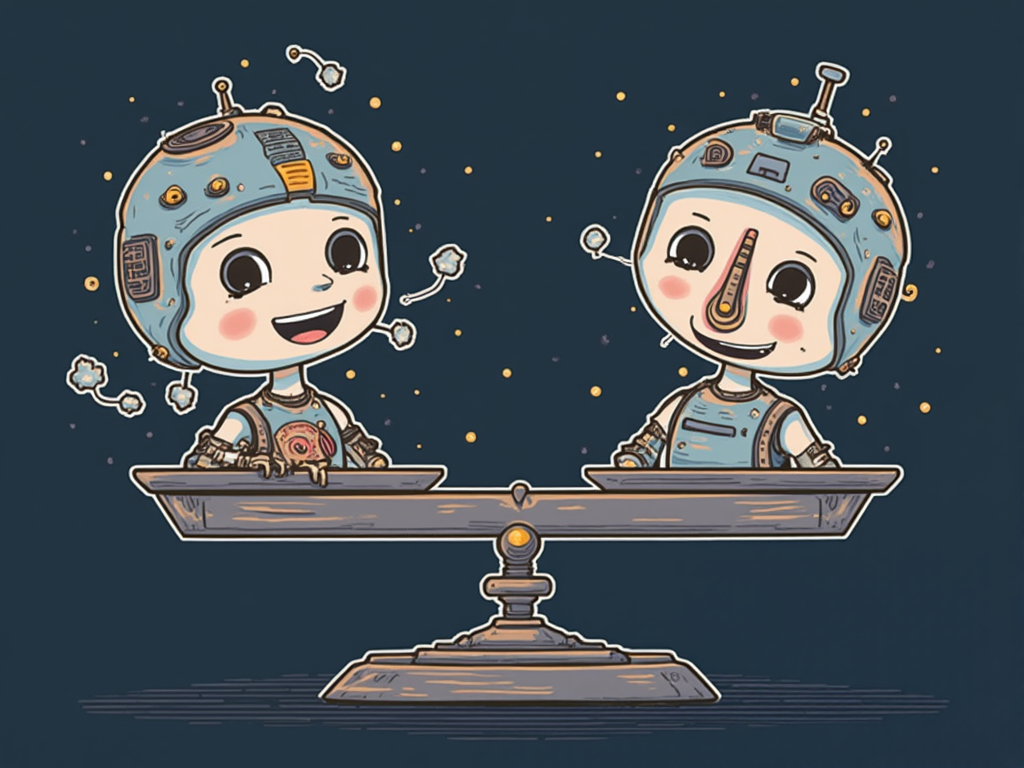